The one-sentence summary
We think we are better at prediction than we truly are, so we need to be more modest and use proper maths to establish true probability.
WHAT THE BOOK SAYS 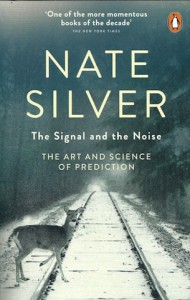
· Prediction is both a science and an art. We routinely fail to foresee hugely significant events. The rise of ‘big data’ has the potential to help us predict the future, yet much of it is misleading and useless.
· The signal is the truth – a piece of evidence that tells us something useful.
· The noise is what distracts us from the truth – random patterns, or confusion produced by competing signals. (The terms originally came from the field of electrical engineering).
· There is positive and negative feedback. In one case, both things go up. In the other, one goes up, the other down.
· Fear and greed balance each other in most markets.
· Most failures of prediction stem from a false sense of confidence.
· Accuracy and precision are often confused: a prediction has to be both to be of any value.
· There are two types of people who make predictions:
Hedgehogs. They believe in big ideas and governing principles. The way they think is specialised, stalwart, stubborn, order-seeking, confident and ideological. They make for good TV but are rarely accurate.
Foxes. They are more scrappy and believe in a plethora of little ideas and methodologies. The way they think is multidisciplinary, adaptable, self-critical, tolerant of complexity, cautious and empirical. They are right more often. (These typologies are based on an essay by Isaiah Berlin.)
· There are three important principles for decent prediction:
1. Think probabilistically (that’s maths, not random prediction).
2. Predictions should be changed in the light of new information.
3. Look for consensus (group forecasts are 15-20% more accurate).
WHAT’S GOOD ABOUT IT
· Overfitting is arguably the most important scientific problem you’ve never heard of. This is when an overly specific solution is provided to a general problem – fitting the noise in the data rather than discovering its underlying structure.
· Bayes’s theorem is concerned with conditional probability, based on events that have already happened. The equation takes a hypothesis, applies an initial estimate of what might happen (a prior probability), establishes the probability of it being true or false, and generates a posterior probability (revised estimate). This approach makes predictions less and less wrong.
· Calibration punishes overconfidence. It is the number of times a forecast turned out to be right. This learning is used to improve the model.
WHAT YOU HAVE TO WATCH
· There are whole chapters on quite specific topics such as US politics, sport, and poker, which may be of marginal interest for non-Americans.